Analyzing Trends: Clustering from Bank Central Asia (BBCA) Historical Close Prices.
01
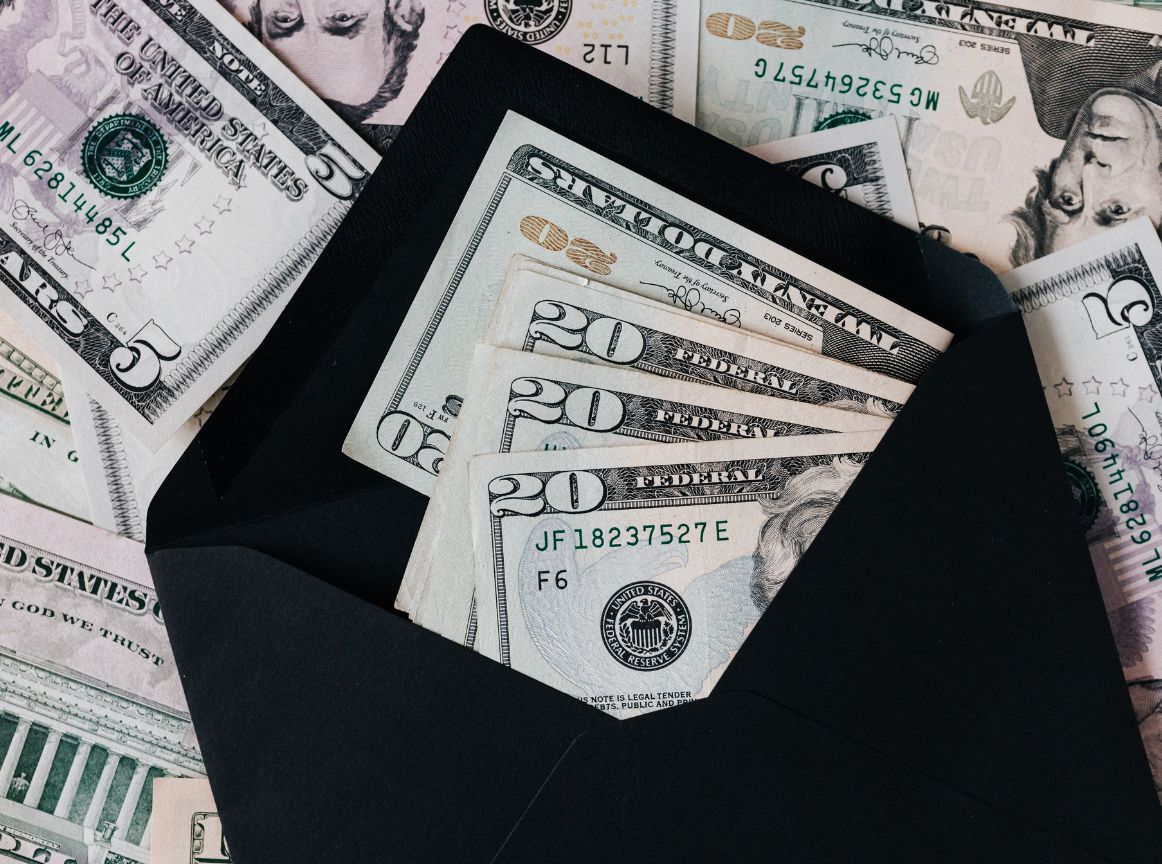
Uncover hidden patterns and insights within Bank Central Asia (BBCA) historical close prices through Markov chain clustering analysis. Explore the intricacies of the financial landscape as clustering algorithms unravel unique clusters.
What is the project?
Embark on a data-driven journey through the financial landscape with clustering analysis of historical close prices in November 2019 - November 2023 from BBCA. Comprehensive study using clustering techniques to unveil hidden patterns and trends within the stock's historical performance. Delve into the intricacies of market behavior, identify distinctive clusters, and gain valuable insights for strategic decision-making. Whether you're an investor, analyst, or financial enthusiast, this exploration of BCA Bank's historical data provides a unique perspective on the evolving dynamics of the market. Uncover meaningful clusters, understand market movements, and refine your financial strategies with this insightful analysis of BCA Bank's close prices over time. Last i would like to extend credit and express my gratitude to Sam Cole and Steve Kirkland for inspiring this project, which also serves as the topic for my thesis. Their contributions and insights have been instrumental in shaping the direction and ideas for my work. I am thankful for their influence in guiding and motivating my efforts.
Research goals:
- The primary goal is to apply the clustering algorithm to the data and extract meaningful clusters. This involves utilizing the algorithm's capabilities for effective data segmentation.
- Investigating and comprehending the techniques and metrics used to assess the quality and effectiveness of clustering outcomes derived from the left singular vector algorithm. This involves identifying appropriate evaluation criteria to gauge the reliability and accuracy of the clustering results.
Conclution:
The clustering methodology can categorize data from stochastic matrix that representing a Markov chain. This categorization was based on the positive and negative values of the left singular vector corresponding to the second smallest singular value. The algorithm iteratively proceeded until the tolerance value was met, and the second smallest singular value satisfied the tolerance criteria for each identified cluster. Clustering results was evaluated using the coupling matrix. he entries of the coupling matrix elucidate the probability distribution of the identified clusters. A higher average diagonal value of the coupling matrix indicates a better clustering outcome.
- Tools: Matlab, Pyton, Microsoft Excel